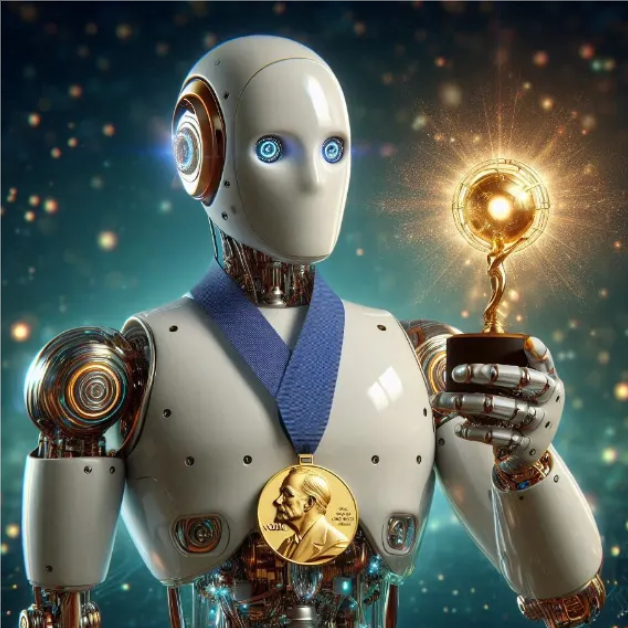
Among the 2024 Nobel Prizes, five laureates across two prizes were honoured for Artificial Intelligence (AI) related research. Specifically, these awards are for research into AI models beyond just generative AI, showing how broad the applications are, how far they have developed and how much further there is to go.
In Physics, John Hopfield and Geoffrey Hinton were awarded the prize for “foundational discoveries and inventions that enable machine learning with artificial neural networks.” Hinton and Hopfield used tools from physics (interestingly, Hinton is not a physicist) “to construct methods that helped lay the foundation for today’s powerful machine learning” according to the Nobel Institute.
“I would be expecting in the next couple of years the first AI designed drugs.” – Sir Demis Hassabis
The prize in Chemistry relates to AlphaFold, the Alphabet (Google) reinforcement learning AI model for predicting protein-folding shapes, resulting in a 1,000-fold increase in the size of the protein data bank from 200,000 to 214,000,000. The recipient, Sir Demis Hassabis, the head of Google’s Deepmind, told the Financial Times that he was “working to expand drug discovery, designing chemical compounds, working out where they bind, predicting properties of those compounds, absorption, toxicity and so on. In a separate interview, he said “I would be expecting in the next couple of years the first AI designed drugs.”
The work of Hassabis and his two fellow researchers is gobsmacking, unlocking a near-impossible problem of overcoming the laborious process of mapping new proteins.
The way in which proteins are “folded” is critical to their function – the stretched out “un-folded” protein may contain all the elements necessary, but without being formed into specific position it may be rendered useless or even worse, fatal. Poorly folded proteins have been implicated in neuro-generative diseases including Alzheimers.
“The impact of [AI] in particular on science but also on the modern world more broadly is now very, very clear,” according to Maneesh Sahani, director of the Gatsby unit at University College London. “Machine learning is showing up all over the place, from people analysing ancient text in forgotten languages, to radiographs and other medical imaging, said Sahani, who is also a neuroscience professor.
“Teaching machines to see”
The Physics prize went to Hinton and Hopfield who built the mechanism through which semiconductors “learn” without actually being coded with a set of specific instructions. The often cited but useful example is of the computer which was shown images, or “trained” on, cats. Once trained the machine is able to recognise cats – despite never having been told what a cat is (the subtlety here is that not all cat images are the same – different colours, different lights, with eyes open or closed, not all parts visible, and so on). This is the foundational stuff by which AI can sort apples by quality, or choose different programs to stream or write sonnets. One of Hinton’s team, Ilya Sutskever, was a founding member of OpenAI.
If there was a moment when markets collectively doubted the importance of AI, these Nobel Prizes should silence some of the noisier critics. Large language models (LLMs) have animated share markets across the last two years. Even though it is the non-LLM models (like AlphaFold) gaining Nobel Prizes, we believe the opportunity presented by these models is not priced (yet). Click here for a deeper dive on this opportunity and the mechanisms that underpin it.
Not only are these models much smaller than LLMs – making them feasible for on device (“edge”) inference – they exist across a much more diverse set of end uses. Non-LLM models include sound processing (voice recognition), robotics, 2-D image recognition (cancer screening detection) and in 3-D object recognition (autonomous vehicles, see our earlier Insight, “Chips are taking the wheel“) and more.
Racing to the “Edge”
Over time, more and better data will be collected, better techniques pioneered and demand for AI will emerge in new fields. If these models can be run effectively on the edge today, then the number of models may increase independent of improvement in datacentre computational power. What has been developed already is a runway for many years of disruption across a diverse set of industries.
Companies are already bringing AI to devices. Apple’s work on its version (Apple Intelligence) and Alphabet’s Gemini Nano models are just one piece of the puzzle. This will push demand for high-end chips like those supplied in phones by Qualcomm and in PC’s by Advanced Micro Devices. Meanwhile these chips, as well as the cloud chips, are largely fabricated by Taiwan Semiconductor Manufacturing Company.
All are holdings in portfolios we manage. We do not hold every AI company – a position in one always comes at the expense of holding another, so valuation decisions are critical if performance is to remain solid.
Share this Post